Software AG Blog Homepage
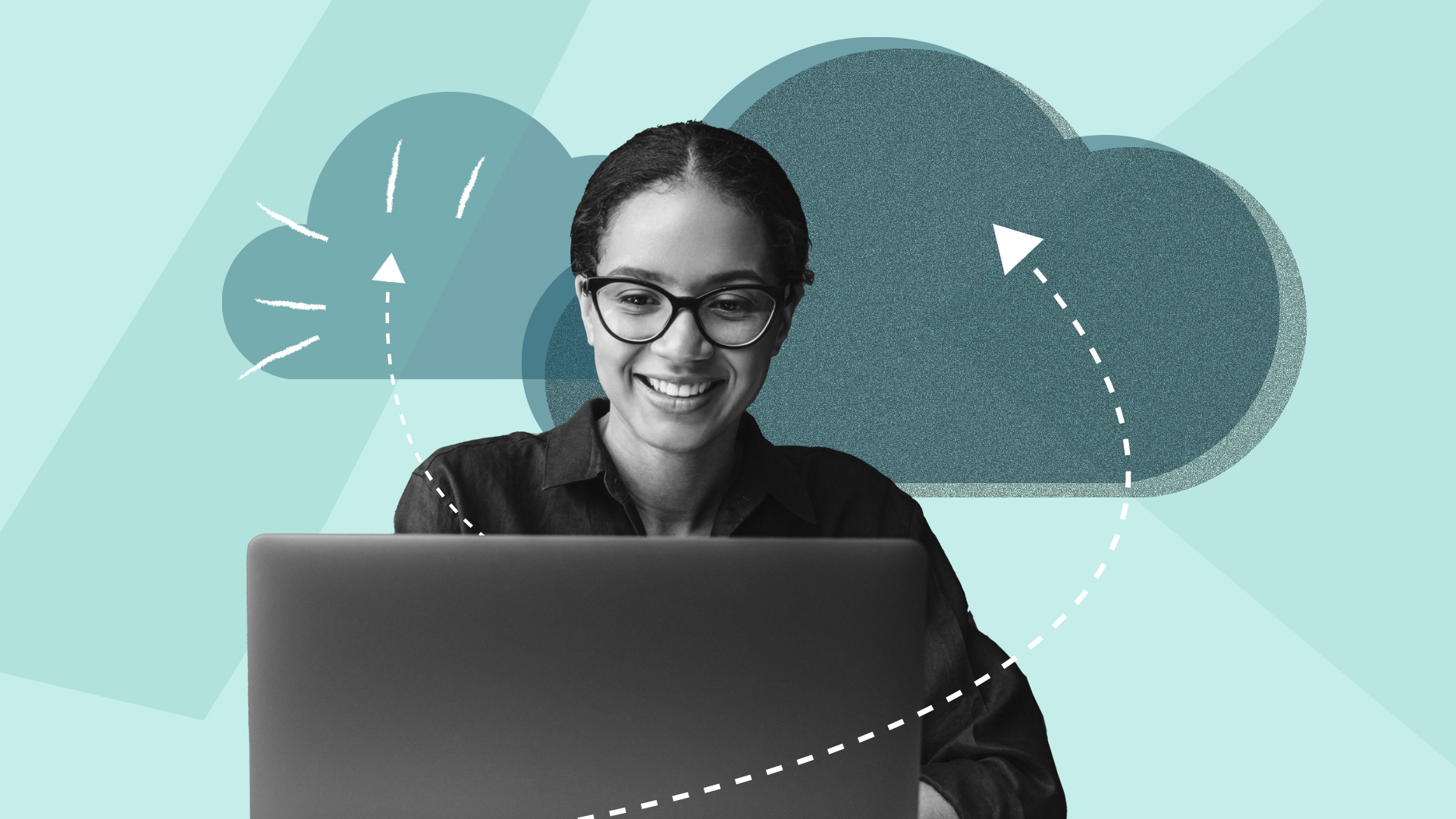
App & Data Integration
Is it Time to Move Your B2B Integrations to the Cloud?
B2B integration may not be the first type of integration you think of when hearing the term, but you’ll be hearing more and more of it in the coming years. The reason for that is simple—traditional B2B integration platforms are…
Read blog
Recent posts
-
Smarter, more sustainable racing
How the ERA Championship is staying in pole position
-
Subscribe now and never miss a post!
App & Data IntegrationMulti-Cloud Integration Simplified: webMethods.io Integration 11
The era of multi-cloud is officially here. With that, the importance of multi-cloud integration is increasingly growing as companies navigate between multiple clouds, integration platforms, and integration patterns. This new chaos of connectivity creates multiple challenges, demanding innovative solutions and…App & Data IntegrationHow the API Control Plane empowers API Product Managers
In October, we introduced Super iPaaS – our vision for the iPaaS category. It cemented our belief that all integration patterns should coexist to deliver new and unprecedented levels of productivity, agility, and governance. webMethods.io API is a key component…App & Data IntegrationThis is how an iPaaS should work—develop anywhere, deploy anywhere
It’s been over a decade since Gartner introduced the Pace-Layered Application Strategy. This model, a way of organizing and managing applications to align them with business goals, is even more relevant today. The idea is that within an organization, there are…Process ManagementCustom Processes: A Cornerstone for Successful Application Lifecycle Management (ALM)
Achieving successful business transformation hinges on the ability to connect various facets within your company into a cohesive whole. Without a well-aligned strategy, business processes, IT (Information Technology) infrastructure, applications, and engagement of all relevant stakeholders, navigating today's economic landscape…App & Data IntegrationIs Integration Dead? Life after AI
In today’s ever-evolving tech landscape, the buzz surrounding artificial intelligence (AI) has never been louder. But what does this mean for the world of integration? Is it on the brink of becoming a relic, or are we just witnessing the…IoTNavigating Your IoT Strategy Amid Microsoft IoT Central Updates
Recently, Microsoft caused a stir by seemingly announcing the imminent deprecation of its IoT Central platform. This sparked concern when an alarming message on the Azure console stated that new IoT Central applications could not be created after April 2024. …App & Data Integration7 Numbers that Define the “Chaos of Connectivity”
In the fast-paced world of IT, numbers often speak louder than words. IT leaders continue to invest in new technology platforms to drive innovation and improve the experience for their customers, employees and partners. (This, according to our annual “Reality…Process ManagementHow to Build Operational Resilience & Fortify Your Enterprise
In the ever-changing landscape of business, uncertainties loom large. From regulatory shifts to global crises, companies face a myriad of challenges that can disrupt operations and threaten their survival. However, amidst these uncertainties lies an opportunity – the opportunity to…App & Data IntegrationBridging the Gaps in LLMs with Your APIs
The ability to create and utilize tools to overcome challenges marks a decisive moment in human evolution, dating back over 3.3 million years. To this day, we remain fascinated by highly intelligent animals that display signs of this ability. Large…NewsIt’s Awards Season, and We’re a 3x Winner on G2’s Best Software for 2024 Awards List
The awards season is upon us. And while we’re as upset as anyone that Barbie’s Greta Gerwig wasn’t nominated for Best Director, we’re focused on the recent G2 announcements. (A G2 award is the “G” in an EGOT, right? Right?) …Process ManagementNavigating the Future of Operational Excellence: Trends for 2024 and Beyond
When we hear about "operational excellence," renowned companies like Toyota and General Electric often come to mind. But what exactly is operational excellence, and how crucial are business processes within this practice? More importantly, what trends in process management and…